How This AI Camera Tech Might Outdo Dedicated Cameras Soon
The AVA AI Robot Videographer is doing what you only dream your camera could do.
When the AVA AI Robot videographer hit Kickstarter, it confirmed a lot of what I’d thought was already possible. A few camera manufacturers are working on improving their AI algorithms. Canon and Sony do a great job already. OM Digital Solutions also some too. Even Leica boasts those capabilities. But understanding how an AI Camera works is even more exciting.
We had a short interview with Paul Jessop, one of the leads of the AVA AI Robot project. His insight into the industry is incredibly fascinating. Some of the main takeaways:
- Google and Facebook open source this info. Folks can use it freely in the development of their own AI Camera tech.
- Tracking balls is pretty simple.
- Horses are pretty simple too.
- Humans are simple, except when they’re curled up like a ball.
- Birds are a bit more difficult. So it’s fantastic that Canon and Sony were able to do it.
- Dedicated cameras with higher megapixel counts have it harder. The less-dense the pixels, the easier it is. Theoretically, this could mean that the Sony a7s III has the easiest time with AI Camera tracking followed by the Canon EOS R6.
As a legally blind man who built one of the largest Photography blogs, this is all fascinating to me. And I think of the implications and possibilities it could one day have for advancing human eyesight. Just think of how it can apply to augmented reality? If you train a pair of smartglasses to recognize your friends, it could probably help someone with limited eyesight. They’d be able to spot their friends in a crowd pretty easily. The possibilities are amazing, wonderful, and exciting to think about!
Phoblographer: Can you talk to us a bit about your background in AI and videography?
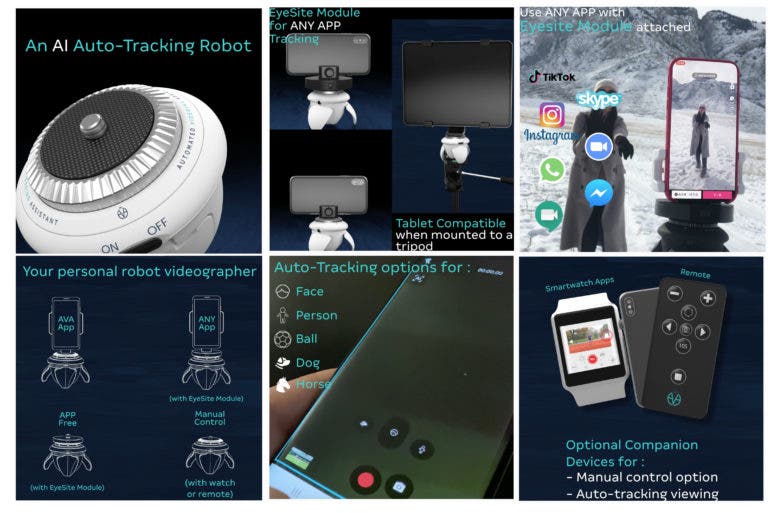
Paul Jessop: My background is in Sport and IT. In terms of education, I embarked on a Master’s Degree Course in Computer Science in 1997 and separately in Computer-Aided Product Design. My time on these courses set me up well for IT and computer science-related future work (albeit ML and AI was very much something of the future back in those days).
Specifically, in terms of AI, my background is just knowledge-based. I have brought in a team specifically on the AI side where their expertise is extensive. I’m more the project manager than on the development team. We need to match a number of niche IT and computer skills in this project from 3D product visualization, product design, electronics, machine learning/computer vision, and motor mechanics.
Phoblographer: Obviously, this requires machine learning and teaching an AI what a body looks like. How good is this technology these days?
Paul Jessop: Technology is very good these days and largely created by the big tech companies (e.g., Facebook and Google) who open-source their technologies for others to expand in their own direction. AVA’s core foundation of ML/Computer Vision platform is based around Google’s open-source machine learning projects called Tensforflow and MediaPipe.
Phoblographer: What sort of factors affect it? Low light? Body shape? Color?
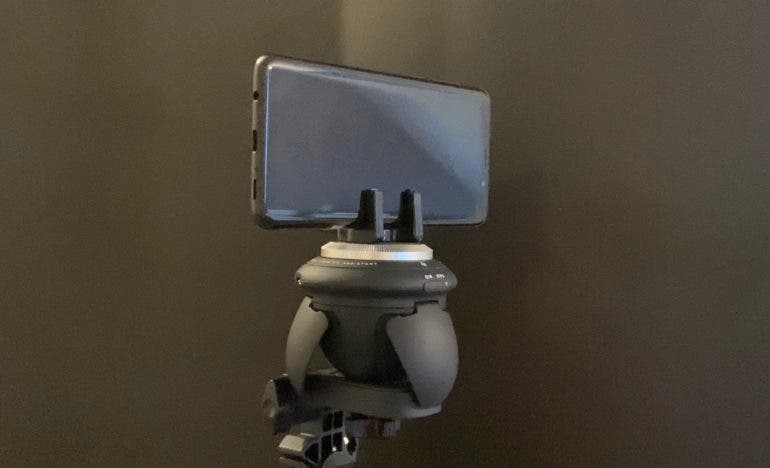
Paul Jessop: Our computer vision model scores an image as a percentage of how certain it is of what it is supposed to be looking for. Typically, it scores a ‘person’ in its view between 70% – 90%. If it is below 60% certainty we’ve coded it to ignore what it is seeing. The same applies to the other tracking subjects (ball, dog, horse, a human face) which are scored in the same way. This is called ‘inference’.
Inference can be affected by body form: for example, if a person is curled up like a ball, the model will be less certain that it is a ‘person’ and will score it less. Equally, if the person is obscured by a large object then this will also reduce certainty. Despite this, the model is still good at recognizing the viewable parts of a person, so even in confusing forms, it will likely still recognize the person in a variety of different non-typical poses and also obscured views. Low light and color don’t tend to affect the inference significantly unless of course, the low light is stopping the images from being seen properly.
Phoblographer: How is it with tracking animals?
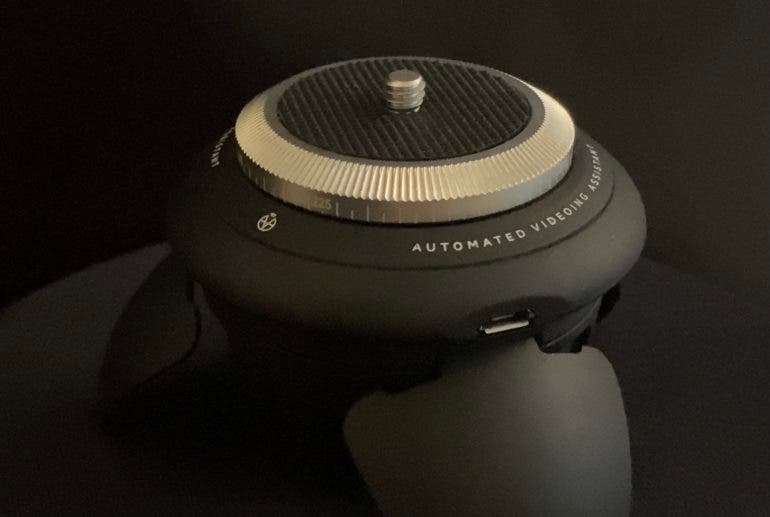
Paul Jessop: Our models can track horses, dogs, birds, and cats. We have only focused on horses and dogs at the moment, and they need quite a lot more optimization before they are usefully useable in our auto-tracking environment. We hope to have this sorted before we ship the devices, but we will be pushing updates ongoing and improving all of the time. As far as cats and birds, we are parking these animals for now whilst we focus on horses and dogs. Birds may never feature on the AVA product as whilst our model can track birds, due to their fast flying speed and movement in the Y-axis, we’re not sure there’ll be much success in auto-tracking birds when we can only move in the X-axis.
Phoblographer: How easily could this be translated over to large sensor cameras? And how effective would it be? Does any of this have anything to do with the deep depth of field a phone gives?
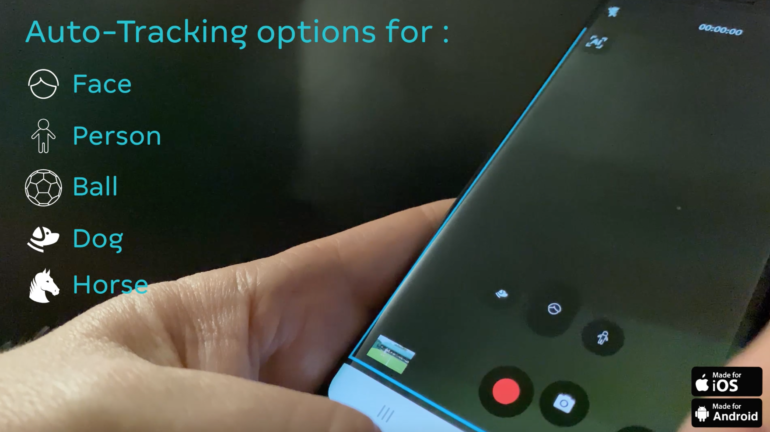
Paul Jessop: Computer vision and machine learning wouldn’t have any extra benefits for large sensor cameras, although they still could be used by them. This is because computer vision works on the pixels in an image and the relationship of those pixels looking like what the model has been trained to recognize. The high sensor cameras offering much greater pixel density would actually take the model longer to identify a person (or other subjects) within the picture because of the greater level of pixels to analyze. Depth of field of a camera also wouldn’t have an effect on the types of models we are using but could be retrained for separate use cases, e.g., trained to recognize clearer pixels compared to blurred effect pixels to give computer vision results.
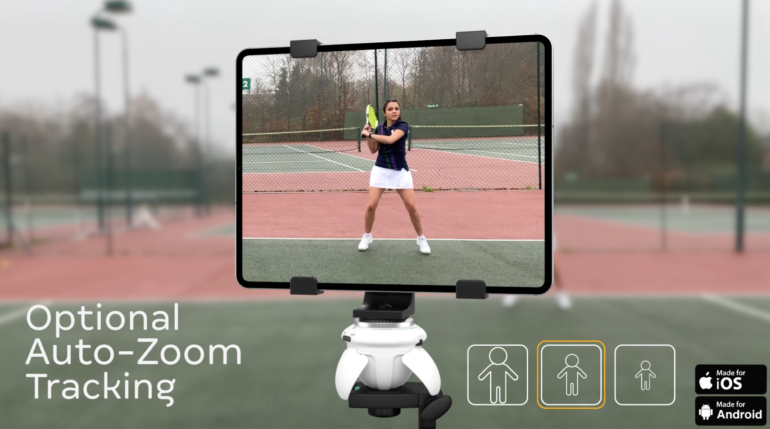
You can pre-order the AVA Ai Robot here. Also, be sure to check out more about the project at their website.